Young Teams grant: Reinforcement learning and planning for large-scale systems
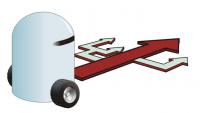
Many controlled systems, such as robots in open environments, traffic and energy networks, etc. are large-scale: they have many continuous variables. Such systems may also be nonlinear, stochastic, and impossible to model accurately. Optimistic planning (OP) is a recent paradigm for general nonlinear and stochastic control, which works when a model is available; reinforcement learning (RL) additionally works model-free, by learning from data. However, existing OP and RL methods cannot handle the number of continuous variables required in large-scale systems. Therefore, this project will develop a planning and reinforcement learning framework for large-scale system control. On the OP side, methods will be developed to deal with large-scale continuous actions and next states. An approach that accelerates large-scale OP by integrating RL will also be designed. The methods will be validated theoretically as well as in applications, with an application focus on assistive robotics. This project is being funded under the Young Teams program of the Romanian Authority for Scientific Research, via UEFISCDI (number PNII-RU-TE-2012-3-0040). It takes place at the Automation Department of the Technical University of Cluj-Napoca, Romania, for a duration of four years and a total budget of about 180 000 EUR.
See also the separate webpage of this project.