Optimal nonlinear control with AI techniques
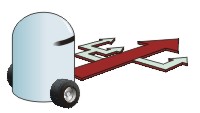
Optimal control can address practical problems appearing in a wide variety of fields, such as automatic control, artificial intelligence, operations research, economics, medicine, etc. In these problems, a nonlinear dynamical system must be controlled so as to optimize a cumulative performance index over time. While optimal solutions have been theoretically characterized starting in the 1950s, computational methods to find them are still a challenging, open area of research.
In this research direction, we focus on the development of methods originating from artificial intelligence and their usage in automatic control. In particular, we are investigating reinforcement learning and planning techniques, developing fundamental lines like complexity analysis on the one hand, and on the other hand adapting the techniques to solve to open problems in nonlinear control, such as networked or hybrid control systems. On the application side, we are investigating the application of these methods to the control of mobile robots for underwater litter collection and domestic assistance.
Our open and ongoing projects in this area are listed below, together with a selection of completed projects where relevant.
